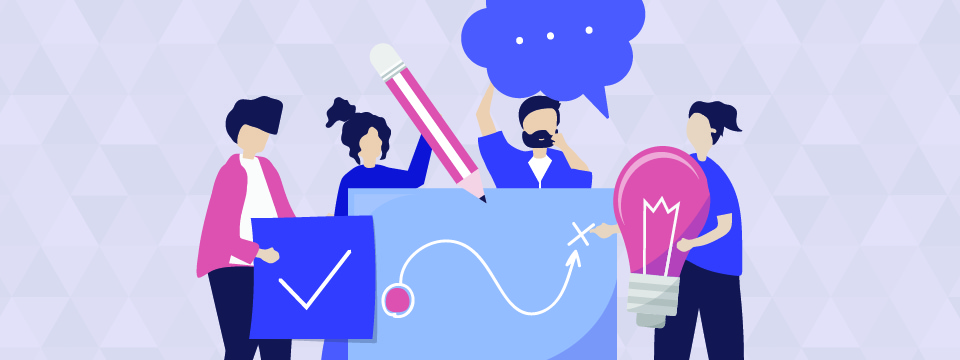
What’s worse? Not knowing you have something or having something you don’t know how to use? Either way, we’re seeing lost opportunities. This logic holds true for anything from a simple kitchen tool to an organization’s most complex data.
Whether you’ve been heating your entire oven for a single piece of toast because you didn’t know you had a toaster tucked away in the garage or you never realized the oddly shaped blade on a handle in your kitchen was actually a vegetable peeler, you’re experiencing inefficiencies in one way or another. The same holds true with data. Without leveraging your organization’s data to mitigate risk (thereby avoiding hefty fines and financial losses) or to generate revenue by predicting customer and employee behavior, you are missing out on the value of what you already have.
Data analytics in the modern enterprise
“Analytics” has been the buzzword for quite some time now. Everyone either promises to do it or wants to. But what can you actually do within “today’s enterprise [which] is hobbled by the lack of the requisite skills in the marketplace, including: advanced statistics/mathematics, new analytics methodologies, advanced systems analysis, business fundamentals, regulatory and legal understanding, and general IT technical and data architecture skills?”
CEO of ZL Technologies, Kon Leong, believes, “to cope with the shortfall in market supply, companies need to better leverage their existing talent”. He suggests building a cross-functional team within your organization, looking for resourceful individuals, and doing the legwork up front to make sure the data you start with is clean and usable.
Building a cross-functional team
A team need not be limited to direct colleagues. Often, we find ourselves in the same circle of coworkers with similar job functions in the same group or department— when indeed branching out to others could allow diversity to increase overall team value.
For instance, Leong suggests that “IT technology and process experts, [who] understand the nature of the data flow and operational processes” work with records managers, “the curators of business records, both physical and digital…[who] know the locations of important documents and how they are categorized and catalogued”.
In addition, guidance must be sought from legal and compliance managers who “understand at a high level what data can be stored, and how they can be used, while minimizing the risk of running afoul of legal and regulatory guidelines”, from regulatory data managers who, “understand which data is retained or deleted due to regulatory obligations, which can increase or reduce capabilities and possibilities in data analytics projects”, and from data protection officers who, “can help analytics stay on the right path and avoid triggering penalties from incoming and expanding privacy regulations.
Looking for resourceful individuals
Looking for those who can simplify the problem and avoid overthinking the solution is more than just efficient; it’s critical to the success of any data analytics initiative. Leong illustrates this resourcefulness with a brief exchange:
“I spoke with one data analyst at a payments processor who claimed to predict riots with a high level of accuracy in the wake of the Ferguson unrest. One would have thought it was from comprehensive analyses of complex demographics and collections of red-flag news indicators of social discontent. It wasn’t. He simply tracked the sales of riot-related components such as crowbars and flammable fluids from major hardware outlets and looked for spikes in purchases that exceeded a certain threshold.”
Individuals like these can save time and cost, the typical culprits any project or initiative failure.
Doing the legwork up front
Once you round up the troops, it’s imperative to ensure your data is clean and useable before setting anything in motion. It’s impossible to produce meaningful analytics without meaningful data, devoid of ROT (redundant, obsolete, and trivial data).
This will require an adequate solution to eliminate duplicates and systematically delete old and unusable data (especially as it pertains to regulatory and privacy concerns) based on rules and policies, which would then eliminate white noise that could generate false conclusions and detract from the value of your analysis.
Using this method, ZL Technologies was able to develop their HR Analytics tool by analyzing “individuals’ inbound communications to assess the frequency of questions and from how high up the organization they came, and then analyze the outbound communications to assess the frequency of answers and to how high up the organization they went.” In presenting the data visually with a relational power graph, “the go-to people lit up like a fireworks display”.
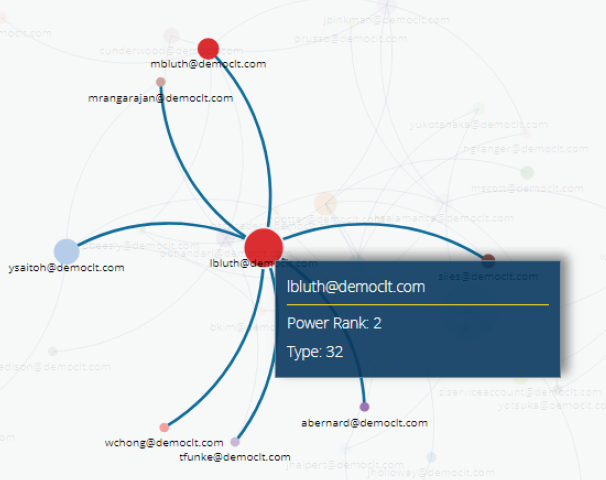
An organization’s success is dependent on leaders, top talent, and key players. So wouldn’t it be nice to know exactly who those are in your organization?
To read more, please visit the original post on Harvard Business Review.
Related Posts
Additional Resources
How to avoid re-processing of data?
Master the Art of Efficiency: Quick Technique on Avoiding Data Re-processing!...
Meta-Data Analysis Vs. In-Depth Analysis
Meta-Data Analysis and In-Depth Analysis are both extensively used by organizations. Here is the comparison between the two approaches and…...
Identifying top performers and key innovators with network analysis
From Anecdotes to Insights - Empowering Organizations with Data Driven Talent Management....